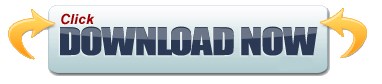

Such datasets make it possible to explore and compare signal acquisition and dynamics, baselining, thresholding, and categorization: this has the potential to identify experimental difficulties and best practice, beyond reproducibility issues.Īmong the various neural signals that support BCI, the availability of fNIRS dataset remains scarce ( Bak et al., 2019), despite its growing popularity, both for BCI ( Naseer and Hong, 2015) and NF applications ( Kohl et al., 2020). Their availability is of particular relevance for applications in which there is significant diversity of practice and lack of standardized protocols, such as Neurofeedback (NF) ( Ros et al., 2020). There is growing interest in sharing datasets for Brain-Computer Interfaces (BCI), to facilitate comparison of technical approaches. Individualized feedback is paramount to the success of NF in BCIs. Beyond providing details of the methodologies used where participants received real-time NF of left-asymmetric increase in activation in their dorsolateral prefrontal cortex (DLPFC), we re-establish the need for carefully designing protocols to ensure the benefits of NF paradigm in BCI are enhanced by the ability of the real-time visual feedback to adapt to the individual responses of the participants. We specifically provide data drawn from our empirical studies in the field of affective interactions with computer-generated narratives as well as interfacing with algorithms, such as heuristic search, which all provide a mechanism to improve the ability of the participants to engage in active BCI due to their realistic visual feedback. We offer the community access to our fNIRS datasets from these experiments. The work presented in this paper is the result of several experiments in the domain of BCI where participants are interacting with continuous visual feedback following a real-time NF paradigm, arising from our long-standing research in the field of affective computing. We accompany our datasets with a detailed description of data formats, experiment and protocol designs, as well as analysis of the individualized metrics for definitions of success scores based on baseline thresholds as well as reference tasks. The subject pool is also drawn from the general population, with less bias toward specific behavioral patterns, and no inclusion of any patient data. From an experimental perspective the BCI context brings more emphasis on individual subjects' baselines, successful and sustained activation during epochs, and minimal training.
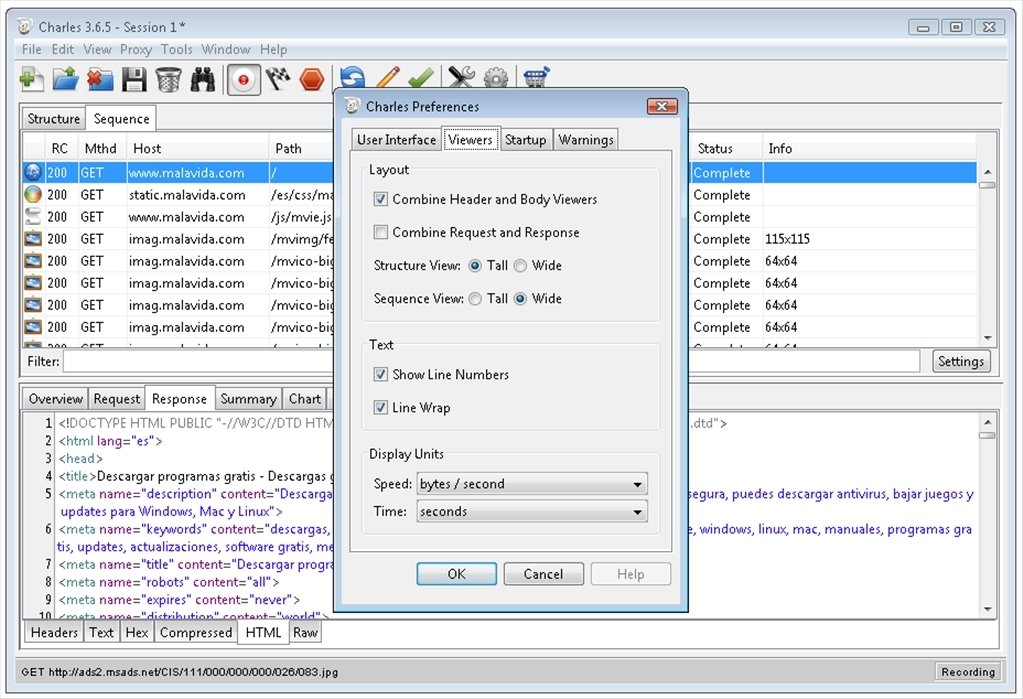
The data presented in this paper are the result of three different experiments using PFC asymmetry neurofeedback (NF) as a Brain-Computer Interface (BCI) paradigm, rather than a therapeutic mechanism aiming at long-term effects, using functional near-infrared spectroscopy (fNIRS) which is known to be particularly well-suited to the study of PFC asymmetry and is less sensitive to artifacts. Prefrontal cortex (PFC) asymmetry is an important marker in affective neuroscience and has attracted significant interest, having been associated with studies of motivation, eating behavior, empathy, risk propensity, and clinical depression. 2School of Computing and Mathematical Sciences, University of Greenwich, London, United Kingdom.1Faculty of Science and Technology, Bournemouth University, Poole, United Kingdom.
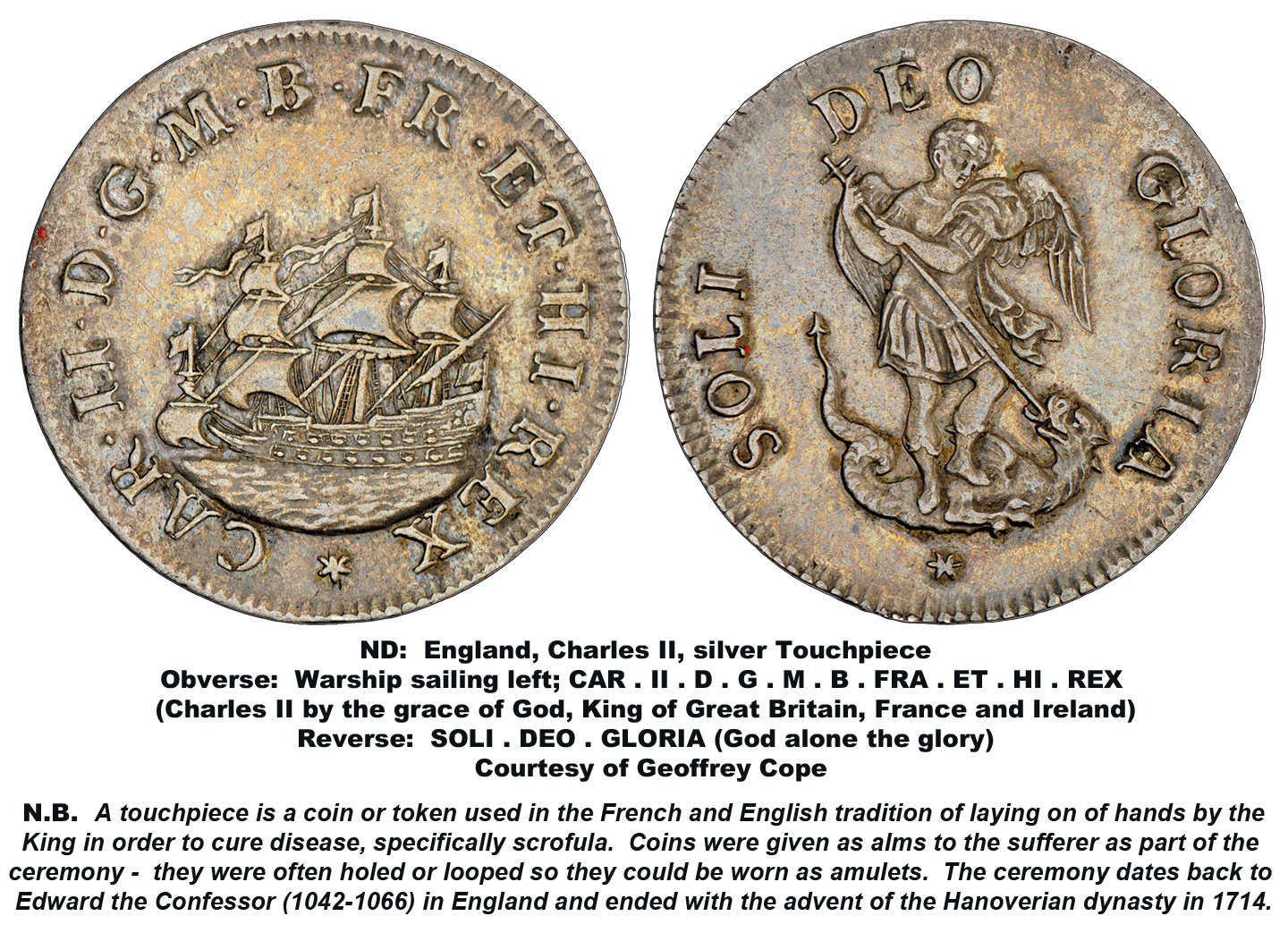
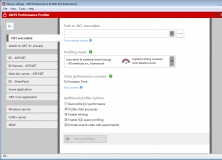
Fred Charles 1 *, Caio De Castro Martins 2 and Marc Cavazza 2 *
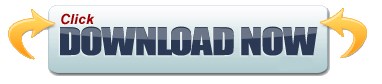